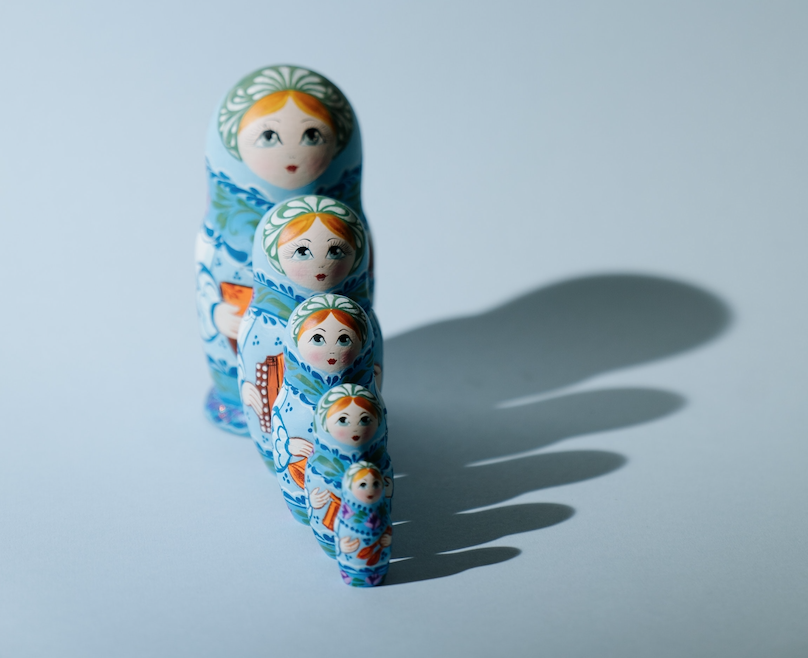
By LARRY SWEDROE
In recent years the field of empirical finance has faced challenges from papers arguing that there is a replication crisis because the majority of studies cannot be replicated and/or their findings are the result of multiple testing of too many factors. For example, Paul Calluzzo, Fabio Moneta and Selim Topaloglu, authors of the 2015 study When Anomalies Are Publicized Broadly, Do Institutions Trade Accordingly? and David McLean and Jeffrey Pontiff, authors of the 2016 study Does Academic Research Destroy Stock Return Predictability? found that post-publication premiums decayed about one-third on average as institutional investors (particularly hedge funds) traded to exploit the anomalies.
Addressing this concern, I explained that the finding that factor premium returns could not be replicated should not be interpreted to mean there is a replication crisis in empirical finance. In fact, they should be entirely expected because institutional trading and anomaly publication are integral to the arbitrage process, which helps bring prices to a more efficient level. Such findings simply demonstrate the important role that both academic research and hedge funds (by way of their role as arbitrageurs) play in making markets more efficient. In other words, lower post-publication premiums do not mean there is a crisis; instead, they show that markets are working efficiently, as expected.
My article then reviewed a March 2021 paper by Theis Jensen, Bryan Kelly and Lasse Pedersen, Is There a Replication Crisis in Finance? Using a Bayesian approach, they examined the claims that findings could not be replicated and concluded:
- The majority of factors do replicate, do survive joint modelling of all factors, do hold up out-of-sample, are strengthened (not weakened) by the large number of observed factors, are further strengthened by global evidence, and the number of factors can be understood as multiple versions of a smaller number of themes.
- While a nontrivial minority of factors failed to replicate in their data, the overall evidence is much less disastrous than some people suggest.
- Using their Bayesian approach, the replication rate relative to the CAPM (the percentage of factors with a statistically significant average excess return) was 85 percent. This was true for both the U.S. and global data (providing out-of-sample tests).
Further evidence
Andrew Chen and Tom Zimmermann contribute to the factor replication literature with their March 2021 study, Open Source Cross-Sectional Asset Pricing. They began by noting that they provided an “open source dataset” of hundreds of predictors of the cross-section of stock returns, allowing researchers to perform their own tests. Their code included 319 firm-level characteristics drawn from 153 research papers. They assigned characteristics to four categories:
- Clear Predictor: The characteristic was expected to achieve statistically significant mean raw returns in long-short portfolios (t-stat > 2.5 in a long-short portfolio, monotonic portfolio sort with 80 bps spread, t-stat > 4.0 in a regression, t-stat > 3.0 in six-month event study).
- Likely Predictor: The characteristic was expected to achieve borderline evidence for the significance of mean raw returns in long-short portfolios (t-stat = 2.0 in long-short with factor adjustments, t-stat between 2.0 and 3.0 in a regression, large t-stat in three-day event study).
- Not-Predictor: Expected to be statistically insignificant in long-short portfolios (t-stat = 1.5 in long-short, t-stat = 1.0 in a regression).
- Indirect Signal: Only suggestive evidence of predictive power (e.g., correlated with earnings/price, modified version of a different characteristic, in-sample evidence only).
Performing their own test, they found that only three failed to reproduce the original papers’ evidence of statistical significance (t-stat > 1.96) for long-short portfolio returns—one of the three that failed still had a t-stat of 1.93. And their t-stats even matched the originals quantitatively: A regression of reproduced t-stats on hand-collected t-stats found a slope of 0.90 and an R-squared of 83 percent. In addition, they also found that mean returns are nicely monotonic in the predictors.
Chen and Zimmerman also explained that the reason some papers have found much higher failure rates is due to their permissive definition of an “anomaly.” For example, the study Replicating Anomalies by Kewei Hou, Chen Xue, and Lu Zhang (HXZ) analyzed 452 anomalies, but “these were derived from only 240 characteristics — 212 of these anomalies were just different rebalancing frequencies of the 240 basic strategies. And of the 240 characteristics, only 118 showed clear evidence of significance for long-short returns in the original papers.”
In their reproductions, Chen and Zimmerman found that 117 out of these 118 clear predictors achieved t-stats > 1.96, and the remaining predictor had a t-stat of 1.93 [73 out of their 74 accounting-focused clear predictor reproductions succeeded, 40 out of 41 price-focused reproductions succeeded, and 43 out of 44 reproductions succeeded among the remaining categories]. In other words, much of HXZ’s ‘replication failures’ are simply due to misclassification: these ‘anomalies’ never had long-short portfolio significance to replicate in the first place.”
The researchers added that they recognised that their reproduction rates may look high compared to those of McLean and Pontiff (MP). However, the difference is reconciled by the fact that 24 of MP’s predictors have what they described as “borderline evidence of statistical significance in the original papers.”
They concluded that “our open source data is highly consistent with MP’s findings. Like MP, we find that returns decay post-publication but remain positive, and that this decay is stronger for predictors that are stronger in-sample.” They added: “Our paper adds to the evidence that the cross-sectional predictability literature is actually quite credible.”
Importantly, they included the caveat that their study did not address the distinct but related question of whether the literature offers implementable trading profits. Research, including A Taxonomy of Anomalies and Their Trading Costs and Zeroing in on the Expected Returns of Anomalies, has found that the effective bid-ask spread wipes out most of the post-publication returns for a significant set of anomalies.
This last point is why, in our book Your Complete Guide to Factor-Based Investing, Andrew Berkin and I included in our list of requirements that before you consider investing in a factor-based strategy, the strategy must be implementable (the premium must survive transactions costs).
Takeaways
For investors, the important takeaway is that despite the proliferation in the literature of a zoo of hundreds of factors, only a small number are needed to explain the vast majority of differences in returns of diversified portfolios. And those factors hold up to replication tests. In Your Complete Guide to Factor-Based Investing, Andrew Berkin and I suggest that investors could limit their tour of that factor zoo to just the following equity factors: market beta, size, value, profitability/quality and momentum.
Important Disclosure: The information presented here is for educational purposes only and should not be construed as specific investment, accounting, legal, or tax advice. Certain information is based upon third party data and may become outdated or otherwise superseded without notice. Third party information is deemed to be reliable, but its accuracy and completeness cannot be guaranteed. By clicking on any of the links above, you acknowledge that they are solely for your convenience, and do not necessarily imply any affiliations, sponsorships, endorsements or representations whatsoever by us regarding third-party websites. We are not responsible for the content, availability, or privacy policies of these sites, and shall not be responsible or liable for any information, opinions, advice, products or services available on or through them. The opinions expressed by featured authors are their own and may not accurately reflect those of Buckingham Strategic Wealth® or Buckingham Strategic Partners®, collectively Buckingham Wealth Partners. LSR-21-62
Image: Cottonbro via Pexels
LARRY SWEDROE is Chief Research Officer at Buckingham Strategic Wealth and the author of numerous books on investing.
ALSO BY LARRY SWEDROE
Cathie Wood and the risk of disruptive technologies
How to avoid foolish behaviour
Why we hold assets we really should sell
How can we explain momentum returns?
Why do smart people do dumb things?
PREVIOUSLY ON TEBI
ESG: manufacturers are the main culprits
The case for tracking the venerable Dow Jones
The all-important pension question
Advising on life’s big changes
Taking on the dependency problem
Why we hold assets we really should sell
OUR STRATEGIC PARTNERS
Content such as this would not be possible without the support of our strategic partners, to whom we are very grateful.
TEBI’s principal partner is Sparrows Capital, which manages assets for family offices and institutions and also provides model portfolios to advice firms. We also have a strategic partner in Ireland — PFP Financial Services, a financial planning firm in Dublin.
We are currently seeking partnerships in North America and Australasia with firms that share our evidence-based and client-focused philosophy. If you’re interested in finding out more, do get in touch.
© The Evidence-Based Investor MMXXI